Mitigating Risks of AI Hallucinations: A Comprehensive Guide


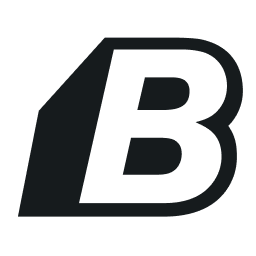

AI (Artificial Intelligence) has revolutionized numerous aspects of our lives, from autonomous vehicles to virtual personal assistants. However, with these advancements come potential risks and challenges. One of the most pressing concerns is the occurrence of AI hallucinations. These hallucinations refer to situations where AI systems produce inaccurate or misleading information, which can have serious consequences. In this comprehensive guide, we will explore the dangers of AI hallucinations and provide strategies for safeguarding against these risks.
The Dangers of AI Hallucinations

AI hallucinations pose a significant threat to various industries, including healthcare, finance, and transportation. When AI systems generate hallucinatory information, it can lead to incorrect diagnosis in medical settings, unreliable financial predictions, and even accidents caused by inaccurate instructions given by autonomous vehicles. These dangers necessitate a proactive approach to mitigate the risks associated with AI hallucinations.
Understanding the Impact of Temperature on AI Systems
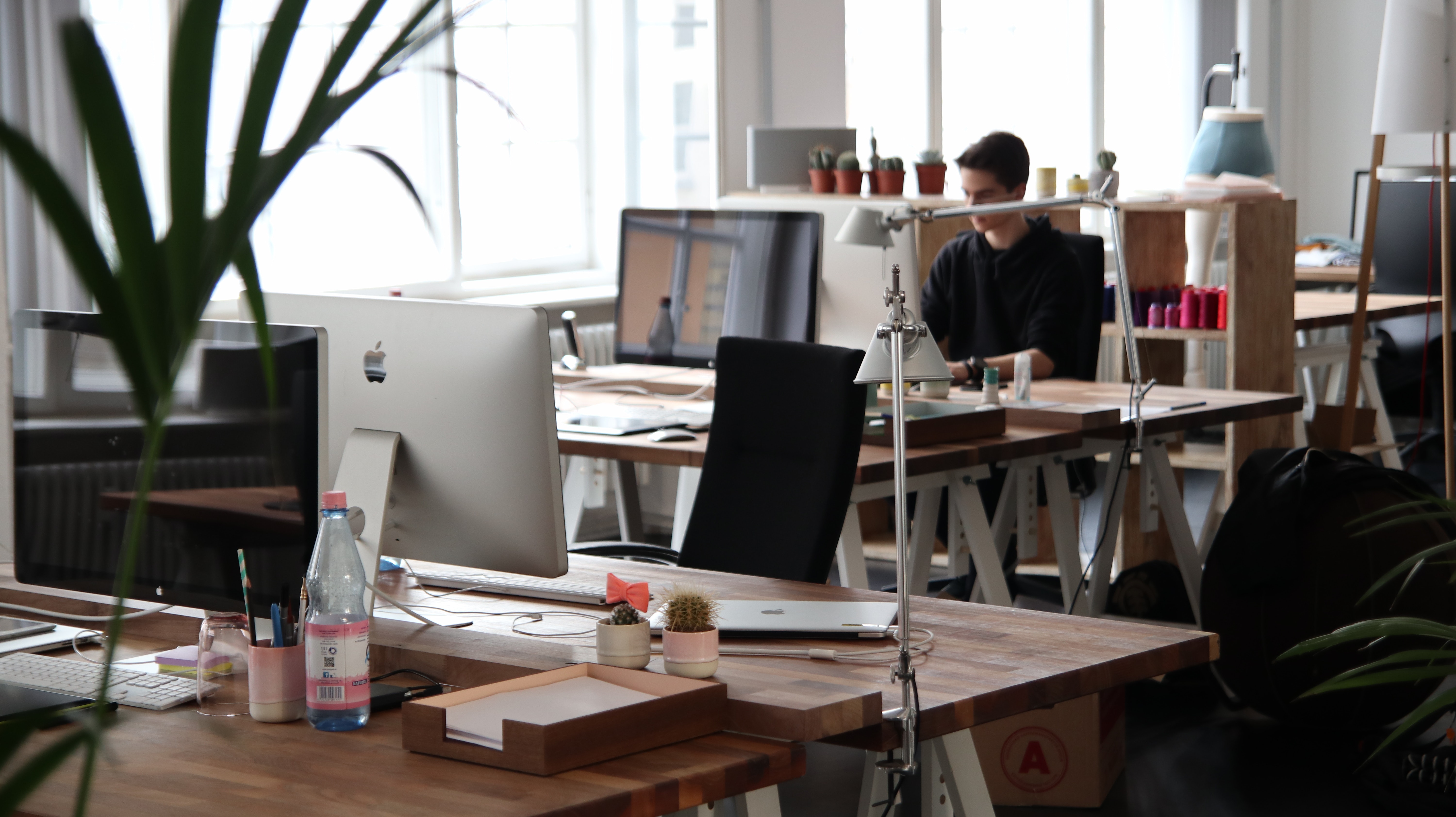
Believe it or not, temperature plays a crucial role in the functioning of AI systems. Extreme temperatures can alter the behavior of AI algorithms, leading to hallucinations. High temperatures, for example, can cause AI models to generate nonsensical outputs, while low temperatures can result in false positives or negatives. To reduce the risks of AI hallucinations, it is essential to regulate the temperature of the hardware and infrastructure supporting AI systems.
When AI systems are exposed to high temperatures, the heat can cause the components to malfunction, leading to unexpected behavior. This can result in hallucinations where the AI generates inaccurate or nonsensical information. On the other hand, extremely low temperatures can slow down the processing speed of AI algorithms, leading to delayed responses and potential errors in the generated output. By maintaining optimal temperature conditions, AI systems can operate efficiently and minimize the occurrence of hallucinations.
Safeguarding Against AI Hallucination Risks
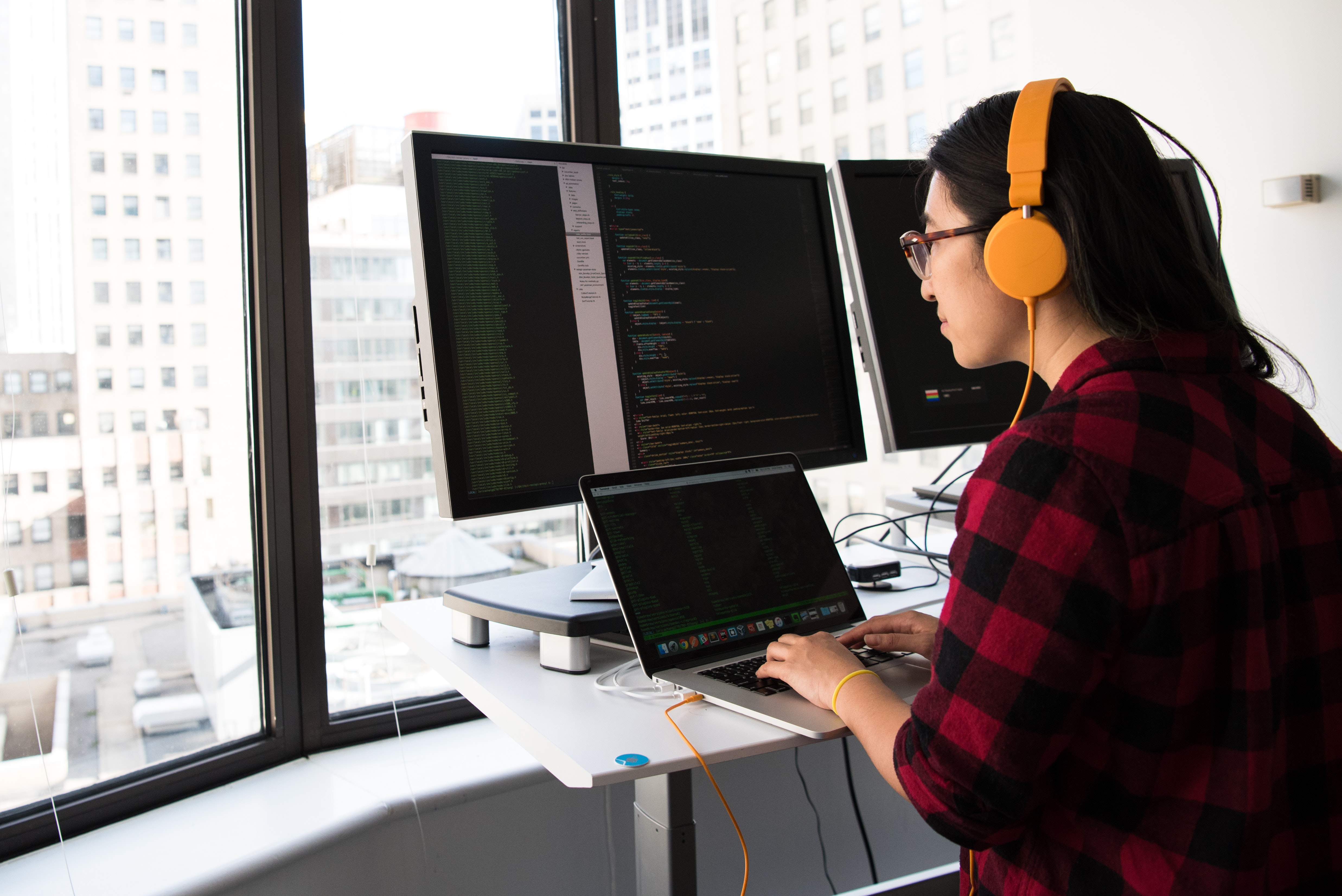
The risks associated with AI hallucinations can be mitigated through a combination of rigorous testing, robust validation mechanisms, and continuous monitoring. Implementing thorough testing protocols ensures that AI systems are subjected to varying inputs and scenarios, allowing developers to identify and address potential hallucination triggers.
During the testing phase, AI models are exposed to a wide range of data inputs, including both normal and abnormal scenarios. This helps simulate real-world conditions and enables developers to observe how the AI system responds. By analyzing the system's behavior under different circumstances, developers can identify patterns that may lead to hallucinations and make the necessary adjustments to improve accuracy and reliability.
In addition to testing, implementing robust validation mechanisms is crucial in safeguarding against AI hallucination risks. Human oversight and validation can help detect and rectify any inadvertent hallucinations. By having human experts review the output generated by AI systems, potential errors or hallucinations can be identified and corrected before they cause any harm or misinformation.
Continuous monitoring is another essential aspect of mitigating AI hallucination risks. By constantly monitoring the performance and behavior of AI systems, any deviations or anomalies can be detected promptly. This allows for immediate intervention and corrective measures to be taken, ensuring that the AI system operates reliably and minimizes the occurrence of hallucinations.
Addressing Bias in AI-Generated Content

Bias in AI-generated content is another considerable concern that affects industries such as media, marketing, and education. When AI systems are trained on biased data, they can perpetuate stereotypes, discrimination, and misinformation. In order to maintain fairness and accuracy, it is essential to employ strategies that mitigate bias in AI-generated content.
One of the most pressing challenges in the field of AI is the issue of bias. Bias can creep into AI algorithms and models in various ways, leading to skewed and unfair outcomes. This is particularly problematic when it comes to AI-generated content, as it can have far-reaching consequences on individuals and society as a whole.
One effective approach to mitigating bias in AI-generated content is diversifying training data. By incorporating a wide range of perspectives and sources, developers can ensure that AI systems produce content that accurately represents different demographics and viewpoints. For example, when training an AI system to generate news articles, including data from diverse news outlets with varying political leanings can help reduce bias and ensure a more balanced representation of different perspectives.
However, diversifying training data alone is not enough. Ongoing monitoring and auditing of AI-generated content are essential to identify and rectify any instances of bias that may arise over time. This involves regularly evaluating the output of AI systems and comparing it against established standards of fairness and accuracy. By doing so, developers can proactively address any biases that may emerge and make necessary adjustments to the underlying algorithms or training data.
Transparency and accountability are crucial in addressing bias and promoting trust in AI systems. Users and stakeholders should have access to information about how AI systems are trained, the data used, and the steps taken to mitigate bias. This transparency allows for independent scrutiny and ensures that AI-generated content is held to high ethical standards.
Furthermore, it is important to involve diverse voices and perspectives in the development and deployment of AI systems. By including individuals from different backgrounds and experiences in the decision-making process, the likelihood of bias being introduced or perpetuated can be significantly reduced. This can be achieved through diverse teams of developers, rigorous peer review processes, and soliciting feedback from a wide range of stakeholders.
Addressing bias in AI-generated content is an ongoing challenge that requires a multi-faceted approach. It requires a combination of diverse training data, ongoing monitoring and auditing, transparency, accountability, and the involvement of diverse voices. By implementing these strategies, we can strive towards AI systems that produce content that is fair, accurate, and representative of the diverse world we live in.
Overcoming the Pitfalls of Generic AI Content

While AI-generated content can be highly efficient, there is a risk of it becoming generic and lacking originality. Generic content not only fails to engage the audience but also hampers the credibility of the organization using it. Overcoming the pitfalls of generic AI content requires creative and innovative techniques.
Techniques to Ensure Unique and Engaging AI-Generated Content
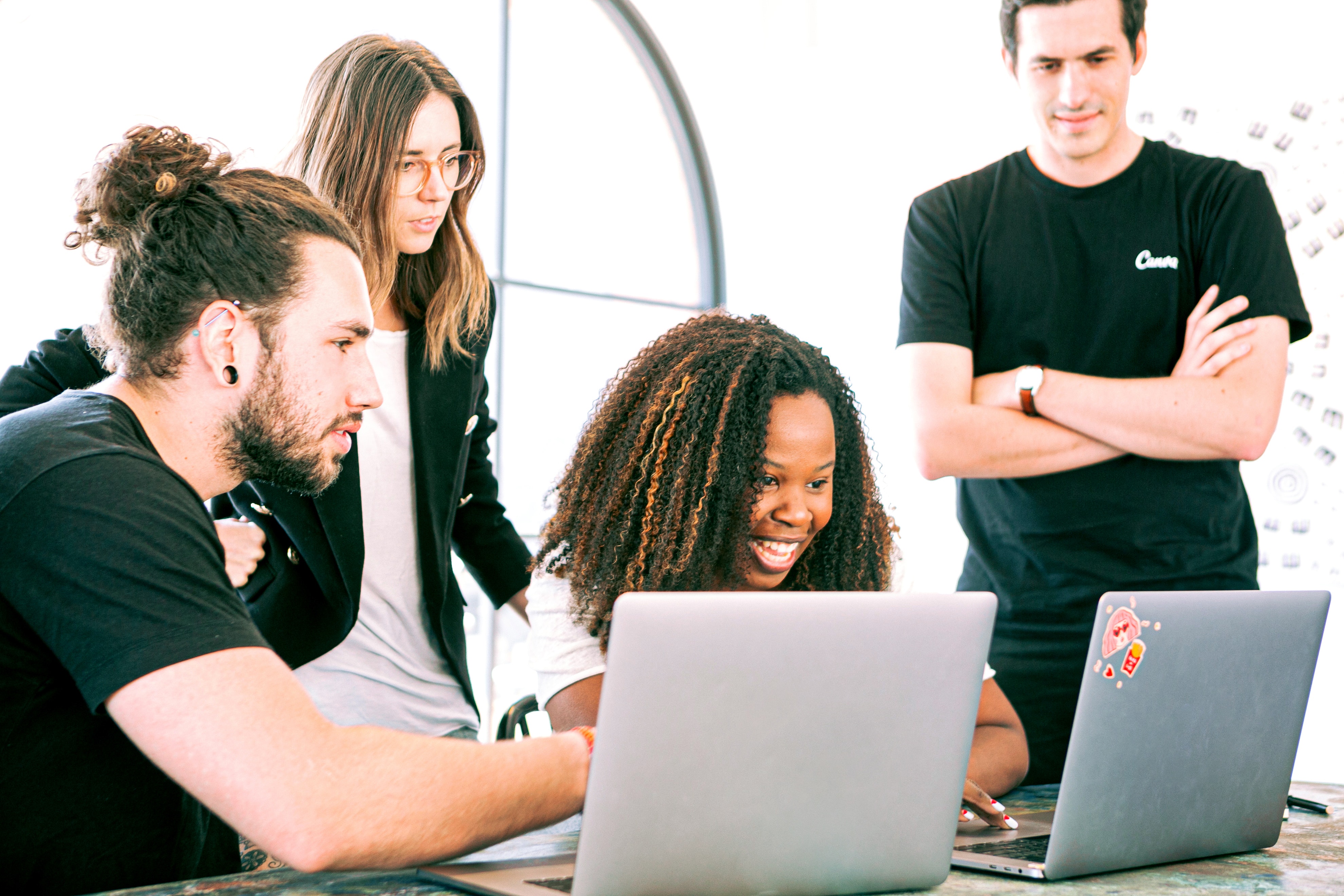
In order to ensure unique and engaging AI-generated content, developers can incorporate techniques such as sentiment analysis and natural language processing. Sentiment analysis helps to gauge the emotional response of the audience, allowing AI systems to tailor content accordingly. Natural language processing, on the other hand, enables AI systems to understand and mimic human speech patterns, resulting in content that feels more personal and human-like. By implementing these techniques, AI-generated content can retain its uniqueness, capturing and retaining the attention of the target audience.
Risk #4: AI's Ethical Implications
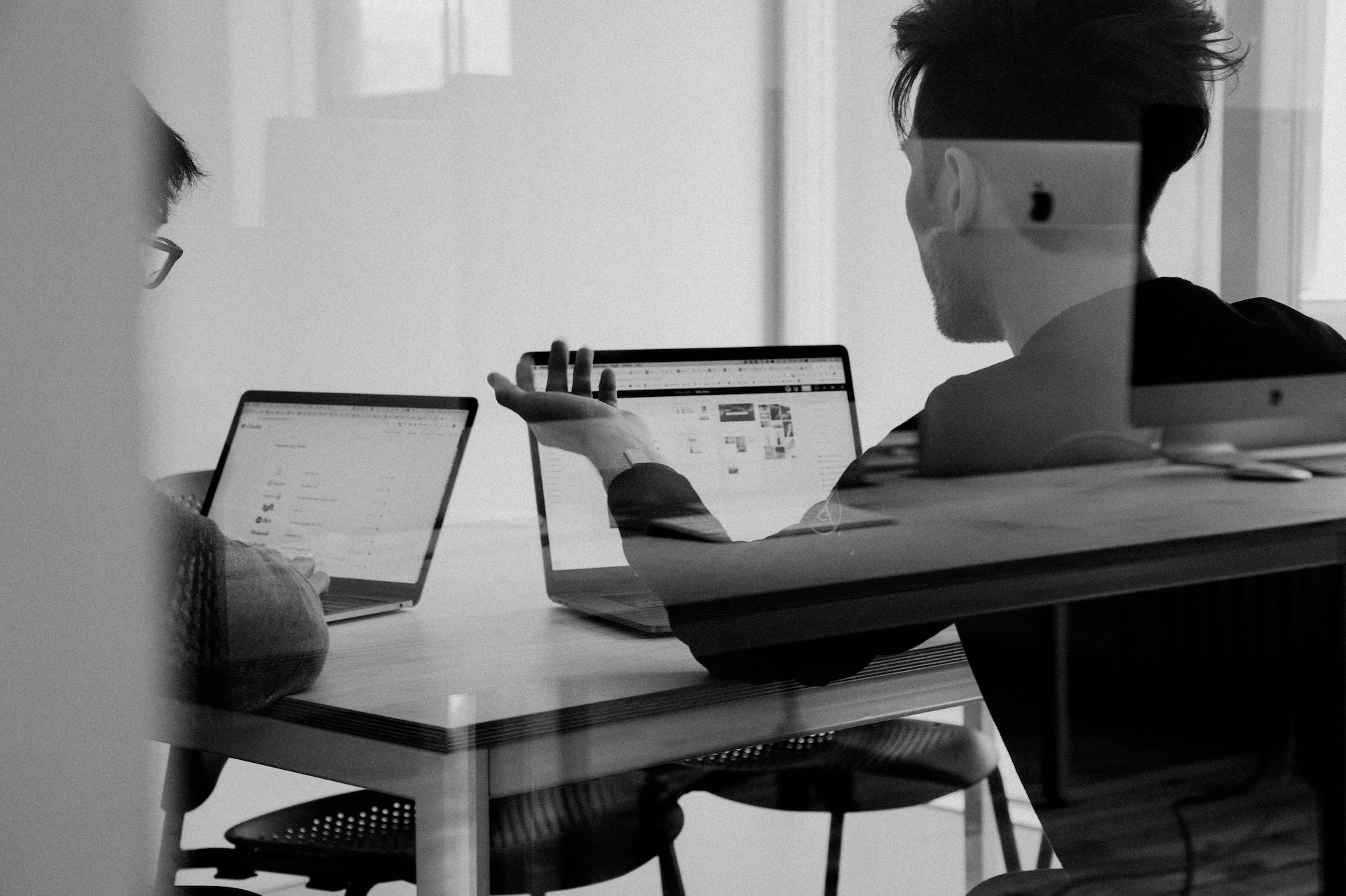
As AI becomes increasingly integrated into our lives, it raises important ethical considerations. AI systems have the potential to reinforce biases, infringe upon privacy, and impact decision-making processes. Understanding and addressing these ethical implications is crucial for responsible AI development and deployment.
Ethical Considerations in AI Development and Deployment
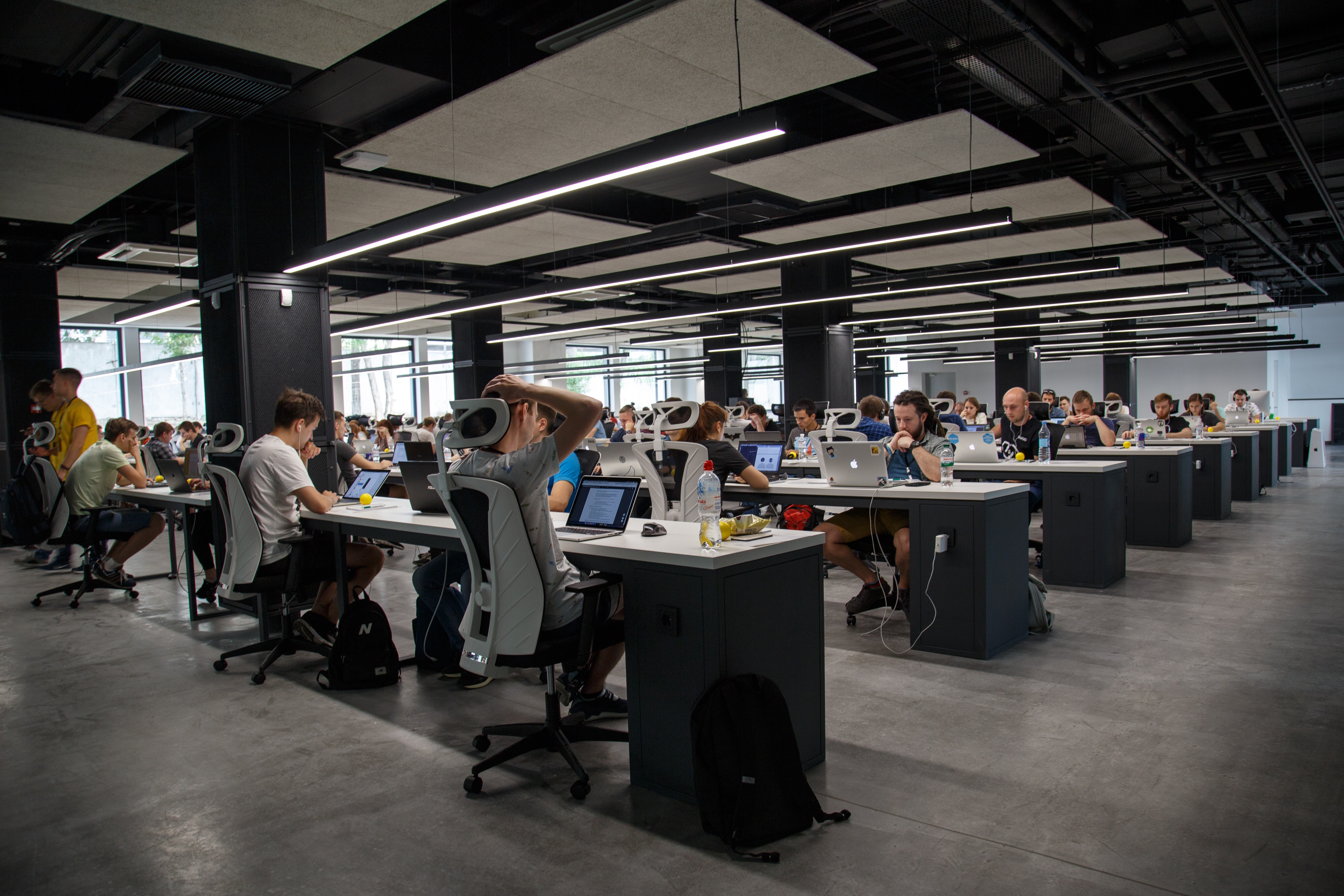
Developers and organizations must prioritize ethical considerations throughout all stages of AI development and deployment. This involves conducting comprehensive ethical assessments, considering potential biases, and adopting frameworks that promote transparency and accountability. By actively addressing the ethical implications of AI, we can foster trust and ensure that these systems are developed and used responsibly.
Ensuring Responsible Use of AI Technology

Responsible use of AI technology requires a collaborative effort between developers, organizations, and regulatory bodies. Implementing effective governance frameworks and regulations helps ensure that AI systems are designed and used in a manner that aligns with societal values and respects individual rights. Furthermore, ongoing education and awareness programs can help users understand the implications and limitations of AI technology, empowering them to make informed decisions.
The Future of AI: Opportunities and Challenges

While there are inherent risks associated with AI, it is crucial to acknowledge the significant opportunities it presents. AI has the potential to revolutionize industries, streamline processes, and enhance decision-making. However, careful navigation of the ethical and legal landscape surrounding AI is essential to fully realize these benefits.
Exploring the Potential of AI in Various Industries

The potential applications of AI across industries are vast and transformative. From healthcare and agriculture to manufacturing and finance, AI has the power to optimize operations, improve efficiency, and drive innovation. Exploring these opportunities encourages collaboration and knowledge-sharing, paving the way for responsible AI adoption.
Navigating the Ethical and Legal Landscape of AI
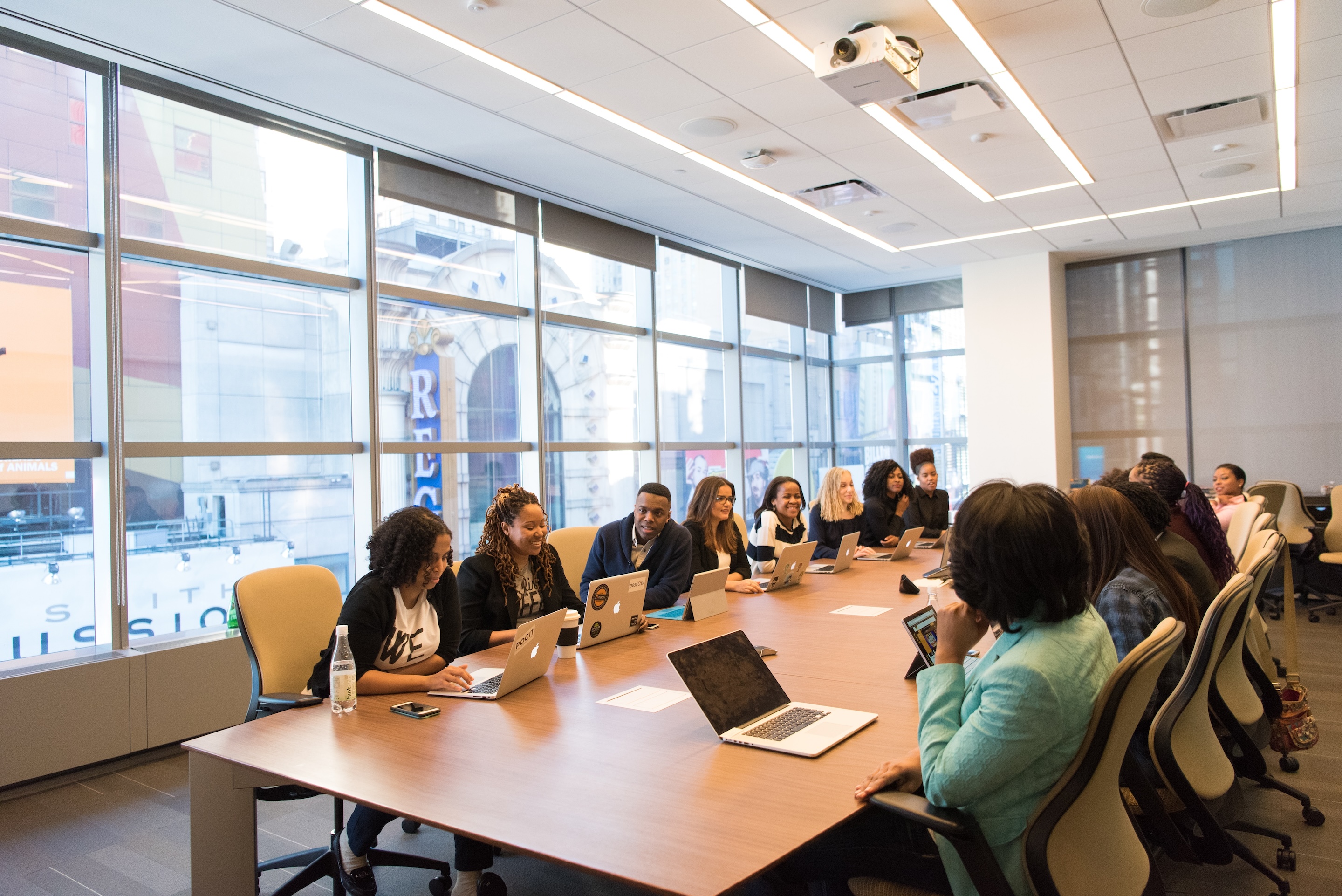
As AI systems evolve, it is crucial to adapt and strengthen ethical and legal frameworks. Addressing concerns such as data privacy, algorithmic accountability, and the impact of AI on employment requires proactive measures. Governments, industry coalitions, and policymakers must work together to establish regulatory guidelines that balance innovation with the protection of societal interests.
In conclusion, mitigating the risks associated with AI hallucinations requires a comprehensive approach. By understanding the dangers, safeguarding against risks, addressing bias, overcoming generic content pitfalls, and considering ethical implications, we can navigate the future of AI more responsibly. Through ongoing collaboration, education, and regulation, we can harness the potential of AI while minimizing its pitfalls. It is imperative that all stakeholders invest the necessary resources to ensure that AI systems are developed and used in a manner that benefits society as a whole.